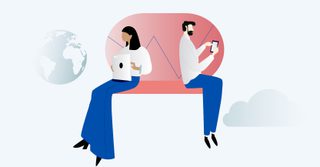
The purpose of corporate data is to allow both management and stakeholders to make decisions and take actions that maximize benefits to the company and to stakeholders. It is a foundational building block to managing and reporting on business-critical issues. Poor data leads to poor decisions and even poorer results. As the popular computer science saying goes: garbage in, garbage out (“GIGO”).
While the digitalization of sustainability (or ESG) data collection, reporting, and management is still in its early days, its deployment will be accelerated by the anticipation and growth of external ESG auditing and investment-grade information requests. To be successful in getting their ESG house in order, companies can leverage experience and best practices in other fields, oftentimes ones that have been developed over decades. One example emerging is financial reporting. Another, more imminent one is data management.
Indeed, if there is a phrase we hear almost as often as “what gets measured gets managed”, it would be “let’s not reinvent the wheel”. And in the case of data management, we certainly don’t need to, because there already exists a body of knowledge, systems, and best practices. Putting the sustainability and ESG lens aside, let’s take a look at the basics of data management and quality.
What is data management, exactly?
Data management is the practice of collecting, keeping, and using data securely, efficiently, and cost-effectively. Technology giant Oracle defines the goal of data management as “to help people, organizations, and connected things optimize the use of data within the bounds of policy and regulation so that they can make decisions and take actions that maximize the benefit to the organization.” Good data management helps ensure that users have trust and confidence in data to be precisely what they expect it to be without the need for manual alignment or data reconciliation.
Data user vs. data owner (which one are you?)
A data user can be a person (stakeholder), an application, or a process that receives and uses data. They determine the characteristics they require of this data and their expectation of quality. They need to know that the data is fit for their intended use.
A data owner is the person with overall accountability for the meaning, content, quality, and distribution of a given set of data. They are accountable for the quality of a given set of data. This includes how the data is defined, manufactured, identified, maintained, delivered, and consumed. It also includes the quality of the data, as defined below.
The seven dimensions of data quality
In the context of corporate sustainability data, the inherent plurality of data owners and users makes quality harder to define and control. Again, we don’t need to look far to understand that quality ESG data is, simply, quality business data.
According to the Enterprise Data Management Council, the quality of data can be evaluated against defined dimensions as well as against the business processes associated with its production. The seven dimensions of data quality are:
- Accuracy: Accuracy is a measurement of the precision of data. It can be measured against either original documents or authoritative sources and validated against defined business rules.
- Completeness: Completeness measures the existence of required data attributes in the population of data records.
- Conformity: Conformity measures how well the data aligns to internal, external, or industry-wide standards.
- Consistency: Consistency provides assurance that data values, formats and definitions in one population agree with those in another data population.
- Coverage: Coverage refers to the breadth, depth and availability of data that exists, and whether it is missing from a data provider.
- Timeliness: Timeliness is a measurement of how well content represents current market/business conditions as well as whether the data is functionally available when needed.
- Uniqueness: Uniqueness refers to the singularity of records and or attributes. The objective is a single source of truth of data.
All of these dimensions are designed to ensure that data is fit for its intended purpose - in other words, good enough to do the job it was designed to do.
Whether or not your business data is ESG-related, its purpose is multifold:
- Measure performance on issues that matter to the business.
- Analyze this performance - over time, against targets, and against peers - to inform the management of these issues.
- Allow stakeholders to make the best-informed decisions (e.g. whether or not to buy the company’s products or to become an employee).
- Enable investors and other capital providers to decide on the most efficient flow of capital towards its intended or desired use, at the appropriate price.
Today, the relatively poor quality and reliability of ESG data remains a significant challenge to its effective use. The digitalization of data collection into a single centralized system of records and the automation of data processing and reporting will go a long way to improving the quality of ESG data, making it easier for it to be used for its intended purpose and to create a virtuous circle of “quality in, quality out” (QIQO).
In Part 2 of this series, we will take a look at how current business processes should support (or could be hurting) your data management ecosystem.