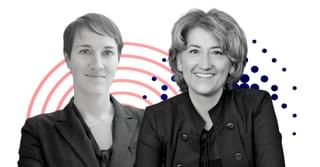
In Part 1 of this series, we described how data management is the practice of collecting, keeping, and using data securely, efficiently, and cost-effectively. We also saw the seven dimensions of data quality: accuracy, completeness, conformity, consistency, coverage, timeliness, uniqueness. Good data management helps ensure that users have trust and confidence in data to be precisely what they expect it to be without the need for manual alignment or data reconciliation.
In this Part 2, we are privileged to have Catherine Nadeau, Senior Manager of Information and Data Governance at KPMG in Canada, as a co-author contributing her subject matter expertise.
What does data quality mean for your organization?
Not all data requires the same level of quality–for example, data for disclosure vs. data used in an exploratory context. We can think of the level of quality in terms of the number of dimensions for which a given data point must be of “good” quality. A data point may be of “good” quality in one dimension but not another. For example, if you put a phone number in the field for a zip/postal code, the data will be at 100% quality for completeness (meaning the field is complete) but at 0% accuracy.
In other words, data quality is context-specific (what is the data’s intended purpose?) and this context determines which dimensions are relevant to use in measuring and determining data quality. Data quality should also be measured and managed throughout its entire lifecycle, because data flows and interaction with other data may alter the level of quality. For example, if, following a transfer to a data warehouse, numbers are truncated rather than rounded after two decimals, their quality may be impaired. You need to implement controls to monitor data quality throughout its useful life, and assign people to remediate quality issues as they arise (this will be covered in more detail in Part 3 of this series).
Data governance goals and objectives
Data governance is more holistic than data management because it is an important business area that requires policy and oversight. Data governance ensures that data is well-managed, used, and disposed of–while data management provides the “how”. Data governance is truly the foundation for quality data.
Like anything else governance-related, data governance should be embedded in your organization’s governance structure–you do not want to create something that is not aligned with your current way of overseeing the rest of your company. The best way to maximize the value of your data governance is by making sure it contributes to the strategy and the objectives of your organization. For example, ensuring the proper definition and the good quality of data serves to improve the reliability and credibility of your reporting; it also serves to improve management functions.
As companies strive to embrace data-centric cultures, we often see data governance as a key component of larger initiatives such as digital transformation or change management. Making your company more data literate requires training your employees, developing their capabilities, and creating a culture where decisions are made based on data rather than gut feelings. This is not easy! A survey of several hundred decision-makers conducted by Wharton Business School at the University of Pennsylvania found that only just 24% of senior decision makers passed the test for data literacy.
Effective data governance is part of a continuous improvement process that requires shifting the cultural mindset and setting up a strong foundation to handle information so that it may be leveraged by the entire organization. This is very similar to the changes involved in embarking on a sustainability journey, and we believe that it should come as no surprise that data quality, digitization, change management, and sustainability are so closely interdependent.
Responsibility and accountability
An important part of data governance is the definition, the documentation, the communication of roles and responsibilities, and, increasingly important, the accountability over data. Since data has become ubiquitous, everyone in an organization is at some point either capturing, accessing, managing, or transforming data. Therefore, everyone has some degree of responsibility over the data life cycle to ensure the proper level of quality.
However, if everyone is responsible for data quality, who is accountable? Legislation around the world, including privacy regulations, are increasingly requiring someone to take on the accountability for data for the organization. For example, GDPR requires a Chief Privacy Officer, and in the province of Québec, the public sector requires a Chef de l’information. Boards are explicitly adding data governance to committee charters—or even creating data governance committees—and the topic is regularly added to the Board’s agenda. Some companies have data governance specialists on staff to support the person acting as Chief Data Officer.
Supporting data life cycle processes through digitization
Collection and capture
Today, data is still often collected manually—this is especially the case for non-financial sustainability-related data. Manual ingestion of data can be done by any number of individuals, and is generally difficult to control or monitor. For large volumes of data, the performance of data entry clerks is usually evaluated based on the quantity/volume they can achieve, rather than on the quality of the data collected. Automating data ingestion through digital tools not only speeds up the process, it also allows for validation controls to ensure the quality of the data along the various dimensions mentioned above.
Access and storage
This protects confidentiality and prevents unwanted modifications, which both contribute to data integrity.
Storage must be adequate to ensure the proper level of security, confidentiality, and integrity, but also to ensure the availability of data. Access controls include logs that keep track of “who accessed what” and “who changed what”. Integrity controls protect data against unwanted alterations or keep track of previous versions. Availability is the capacity to make the data available in a timely fashion to those with appropriate access levels. Compared to physical repositories, digital repositories make retrieving data easier and faster, while better protecting the confidentiality and integrity of that data.
Sharing
Sharing controls and transfer agreements serve to define data ownership and the means by which data is transferred or communicated in a way that protects its confidentiality as well as its integrity, i.e. that no data is lost or modified along the way. This includes any metadata (which is simply data that describes other data). When data is digitized and consolidated in a system of records, it makes it easier to give users access to ESG data than to send multiple copies out to different people.
Retention vs. Disposal
The bigger the dataset, the more difficult it is to store. Whether or not to keep records (and if so, for how long) needs to be clearly defined. While some data assets should probably be kept forever, others should not. This is one (albeit rare) case where technology actually makes things “worse”, as the decreasing costs of data storage and increasing capacity of data analytics result in companies hanging onto their data indefinitely. This is to be avoided, as it increases the noise ratio, gives rise to duplicates, and reduces the certainty of a single source of truth. In some instances, it may even be prohibited by law. For example, companies cannot retain personal data beyond a certain amount of time or they risk considerable fines for doing so.
Closing the loop on data quality and governance
Tying all of this together, the benefits of greater data quality that your organization is likely to reap from digitizing your data life cycle processes are commensurate with the soundness of your overarching data governance strategy.
Part 3 of this series will look at the ways to build the confidence you should have in your data.